Yield Prediction with Satellite Images and Machine Learning
In this project, we propose to classify different plant species in overhead images using supervised machine learning. Monitoring of plants is important for a variety of different applications like protection of the environment, estimation of the acreage per species, and yield forecasting. With an industry partner, we aim at estimating the overall acreage per species for a given area as well as predicting yield.
We rely on powerful deep learning methods, and free satellite images from ESA's Sentinel 2 satellite pair. This constellation offers 10mx10m pixel size on the ground, several infrared and near-infrared channels that are custom-tailored for plant monitoring, and has a revisit time of only 5 days over the same scene increasing chances of acquiring cloud-free images for time-series analysis.
Our first results show that object counting of different tree species at sub-pixel scale is possible. Our methods rely on the spectral signature of each species and the spatial feature of the image to predict the number of trees per pixel.
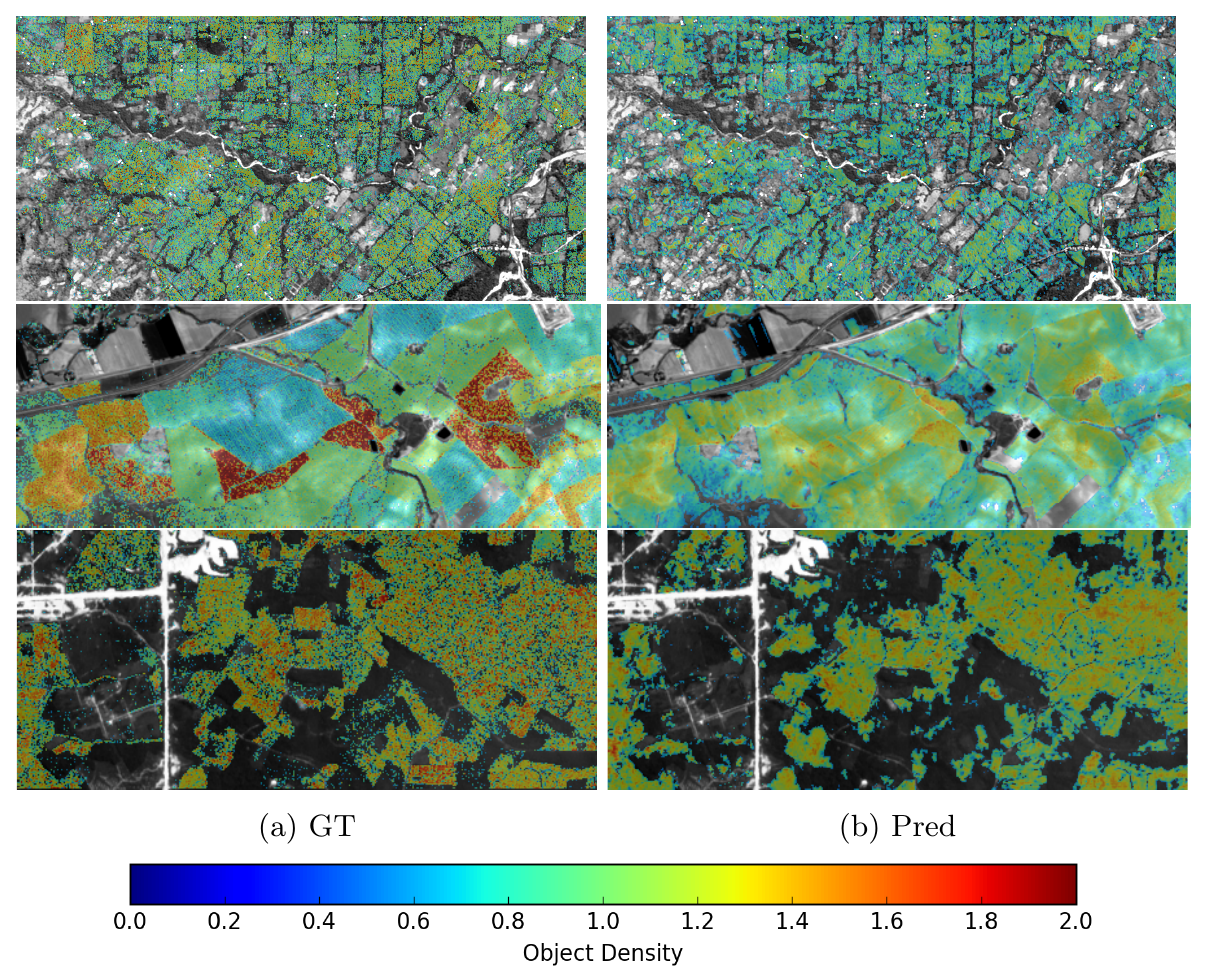
We will further develop a regression method for yield estimation of several species from a time series of satellite images and auxiliary data (e.g., meteorological data).
Much weather data is freely available and can help yield prediction at large scale substantially. Due to frequent cloud coverage, a challenging task will be to make time series analysis robust to irregular imaging intervals. The outcome will be an unbiased, objective, and automated system that uses optical imagery acquired by aerial and/or satellite sensors to estimate tree/crop acreage, and predicts yield.
Publications:
Rodriguez A.C., Wegner J.D.: external pageCounting the uncountable: Deep Semantic Density Estimation from Spacecall_made, German Conference on Pattern Recognition (GCPR), Stuttgart, Germany, 2018
Rodriguez, A.C., D’Aronco, St., Schindler, K., Wegner, J.D.: external pageMapping oil palm density at country scale: An active learning approachcall_made, Remote Sensing of Environment, Vol. 261, 2021
Rodríguez,A.C; Daudt, R.C.; D’Aronco S.; Schindler. K.; Wegner J.D.: Robust damage estimation of Typhoon Goni on coconut crops with Sentinel-2 Imagery. In Review
Contacts:
Jan Dirk Wegner, ETH Zurich,
Andres Rodriguez, ETH Zurich,